A Quick Guide to AI (not just ChatGPT!)
- Atad Data
- Feb 12, 2024
- 6 min read
Since the launch of ChatGPT in November 2022, media reporting on Artificial Intelligence have been dominated by this and other ‘Generative AI’. In fact, broader AI, first defined by Stanford Professor, John McCarthy in 1956 as “the science and engineering of making intelligent machines”, has existed as a concept since ancient times. There are references to intelligent machines more than 3,000 years ago- Homer’s Odyssey refers to self-piloting ships, which ‘navigate by thought’.
.
Most people’s awareness of AI probably came about at some point between the Odyssey and the launch of ChatGPT. The self-piloting ships are pertinent to the driverless car revolution - self-driving cars have been ‘imminent’ for at least the last 10 years, but the final hurdles to become fully self-driving continue to move further away. Autonomous vehicles mainly use a branch of AI known as Machine Learning, and are worthy of a much deeper discussion in themselves, but in this post, we’ll stick to a high-level summary of AI, and some examples of how it can lead to business intelligence.
Data, in its simplest terms, has long been used to make decisions: civilisations learned when and how to plant crops based on previous experience, passed down through generations. And, as discussed in other posts, techniques including statistics and visualisation, have been used by organisations for hundreds of years to better interpret data. The addition of computing power to these analyses meant that they could be done on a much bigger scale.
Many people will have some awareness of how AI is used for things we encounter in real life. Something we can nearly all relate to is email ‘spam’. Once upon a time, we were all bombarded constantly with spam, often selling certain types of products, and very much an unwanted source of a clogged-up Inbox. Depending on your email provider, this should now be less of an issue! These systems have been ‘trained’ on data where the result is known (ie these are spam, these are not spam), which allows the model to flag dubious emails.
As everyone will know, this is not perfect: you will get dodgy emails in your Inbox and you will get legitimate emails in your spam box. If the underlying machine learning model has been trained on representative data, and built and tested well, the false positives (legitimate flagged as spam) and false negatives (spam emails in your Inbox) should be minimised. But, no model is perfect, so there’s a balance to be struck. For what are known as classification problems, this often depends on the scenario: for a business, reducing ‘phishing’ emails is probably worth a few people missing a few legitimate emails. On the other hand, if you’re trying to neutralise explosive devices, and using an ML model to work out which are ‘real’ devices, you’d probably rather blow up a few benign objects than risk missing a dangerous one! You may hear data scientists refer to the precision and recall of a model. This is a good, moderately technical explanation.
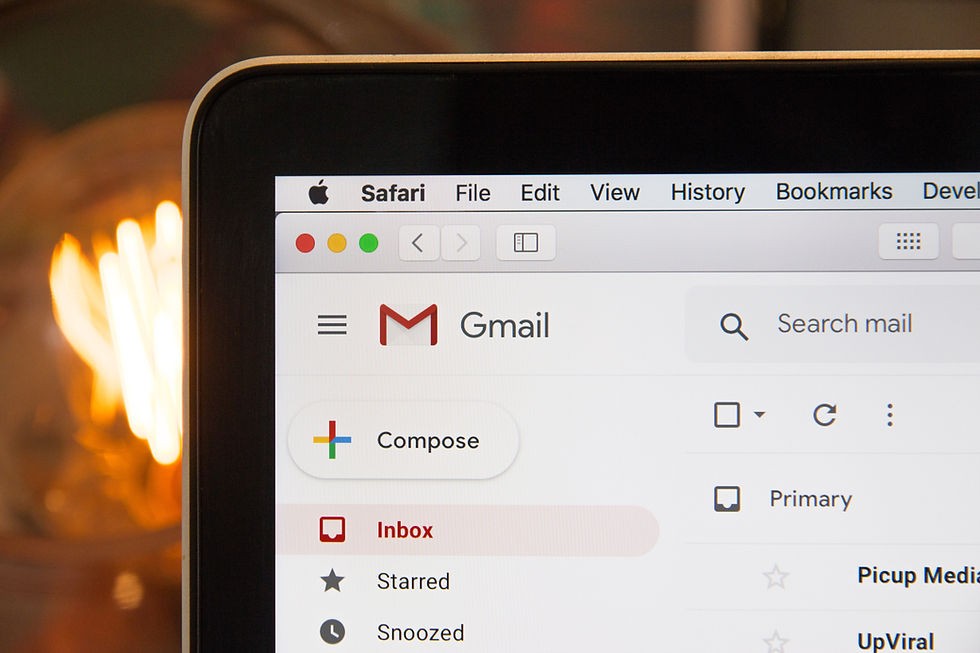
BI has a role to play in this: ideally the model is being re-trained regularly to adapt (eg spammers will keep evolving their methods to evade the model), but if you’re not monitoring and analysing the outcomes of the classification and looking for anomalies and shifts, an out-of-date model creates risks.
In Business Intelligence, we often use time intelligence to monitor historic trends. Time series models have many applications - literally we use them to look forward instead. Although basic seasonality and various types of moving averages can give us some useful forecasts, time series modelling can help isolate other causes of fluctuations and improve these predictions. Some of the most complex examples in this field are weather and stock market forecasting.These approaches massively enhance our predictive analytics capabilities.
In marketing, analysis of customer data can allow customer segmentation. For example, a simple view of customer age group and location might help you identify customers where you make a profit, and customers where you make a loss. However, it remains simple for a reason. Firstly, you need to categorise your data: this takes time (unless you’ve already built the dimensions in your BI system), and even then, you only have these dimensions (features in the ML world), and you’ll need lots of multivariate analyses to find trends. Clustering algorithms allow you to scale up both the data and features. Added to this, they can find ‘hidden’ features you didn’t know about. Using these clusters to segment your data can then also allow further analysis, as well as marketing and other opportunities.
We’re now well used to seeing ‘because you liked this, you might like this…’ on social media, ecommerce, and video and music streaming channels. These work using a variety of recommendation algorithms to suggest based on yours and others activities. For smaller scale organisations, there may not be enough traffic/interaction with your own channels to create your own recommendation system, but it’s useful to have an idea how they work! Where there is scale, these recommendations can also be fed back in to your BI to measure impact.
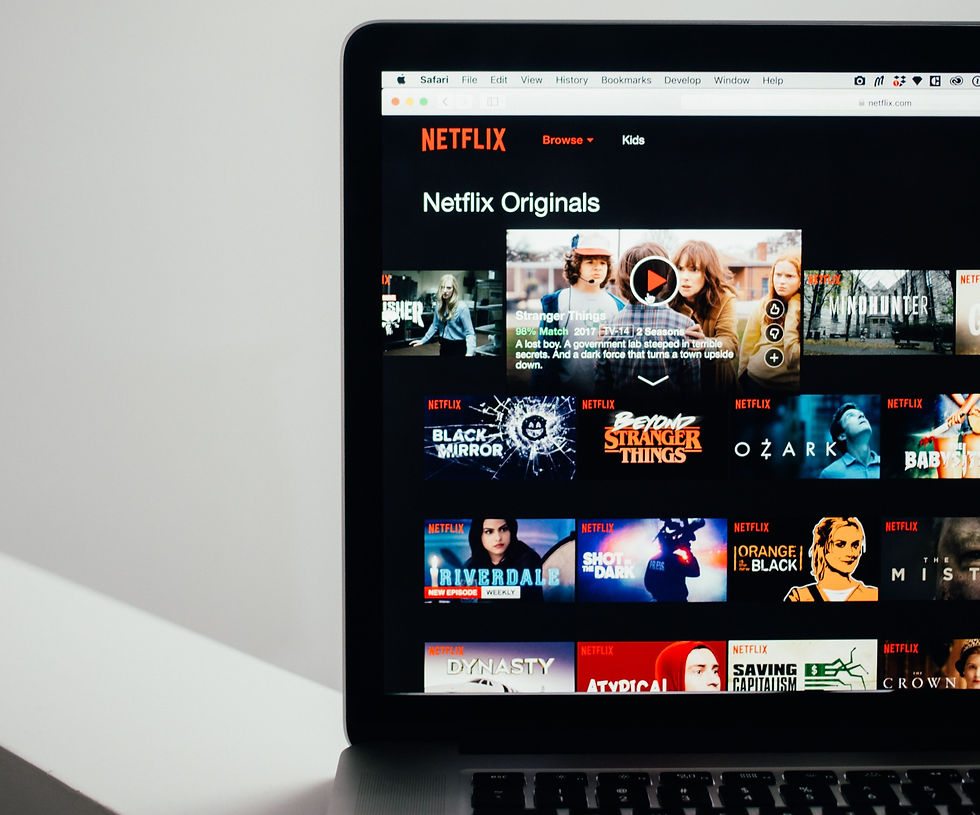
Anyone who’s ever created a survey will know to limit free text answers as much as possible (even if they had to learn by sending one survey out with lots of free text options). Although there are some basic analysis techniques to draw insights from free text (eg word clouds, identifying and flagging specific words), often the text will require some kind of manual classification. Machine learning to interpret unstructured data has massive benefits from a BI perspective. Back to our survey example: you’ve learnt from experience to ask for scores in employee surveys: happiness, feeling listened to etc, but what if you give them a chance to write what they feel, and it tells a different story? Similarly with reviews of products or services- on a large scale, it might be hard to pick out themes without reviewing them all, and crucially, it might be hard to flag complaints that need urgent attention. Classification of and sentiment analysis of text data is somewhere that out-of-the-box analysis tools could start to provide huge benefits.
There isn’t really scope for an explanation of neural networks and deep learning here, but some of the instances where they are used are at the cutting edge of AI. Many people will have seen news reports of ‘deepfake’ videos. By using photographs and a small amount of video and audio of a person, AI can be used to create plausible, but fake videos. An example more likely to be relevant to businesses is speech analytics.
Historically, data from customer service phone calls would be recorded in systems as categorised data, and free-text notes. Like customer surveys, the latter was hard to analyse. However, by transcribing and analysing the call itself (for example to check for consents, themes and sentiment), and using audio signals (eg tone, pace etc), much more can be gained. Speech analytics can lead to great improvements in customer service, and allow issues to be picked up much faster than screening sample calls alone. That is if calls continue to be made by humans at all… there are now AI systems that can ‘converse’ with customers in real time!
Finally, we move on to the hype… Generative AI… There are big questions here. When ChatGPT was first released, it amazed everyone with its ‘creative’ ability. We’ll discuss some of the things it excels at in further articles, but there was one thing it seemed to be very bad at: Maths. From a BI perspective, this seemed pretty worrying. If it couldn’t sort a list of 10 values correctly, how could it tell you about your organisation’s performance? At the time, there was a good explanation for this- Large Language Models are exactly that… language models. Asking it a Maths question was equivalent to asking a class of students a simple multiplication question, then asking a student who knows nothing about Maths to guess a the answer to a new question - they would be guessing based on previous students’ answers without the underlying knowledge, and would have a much better chance of answering if the question was a repeat of that given to another student.
The rapid evolution has solved this pretty quickly: incorporating python code-building ability means that tools such as ChatGPT can solve complex Mathematics. Whether these tools can replace analysts or provide an easy to use tool for non-data people to gain insights on data is a more nuanced question, which will be covered in detail in further articles